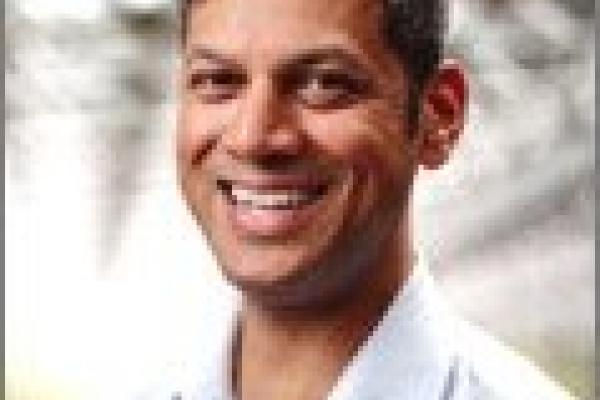
Abstract: Suppose we have many copies of an unknown n-qubit state rho. We measure some copies of rho using a known two-outcome measurement E_1, then other copies using a measurement E_2, and so on. At each stage t, we generate a current hypothesis sigma_t about the state rho, using the outcomes of the previous measurements. We show that it is possible to do this in a way that guarantees that Tr(E_i sigma_{i-1}) differs from Tr(E_i rho) by more than epsilon at most O(n/epsilon^2) times. Even in the “non-realizable” setting—where there could be arbitrary noise in the measurement outcomes—we show how to output hypothesis states that do significantly worse than the best possible states at most O(sqrt(Tn)) times on the first T measurements. These results generalize a 2007 theorem by Aaronson on the PAC-learnability of quantum states, to the online and regret-minimization settings. We give three different ways to prove our results—using convex optimization, quantum postselection, and sequential fat-shattering
dimension—which have different advantages in terms of parameters and portability.
Joint work with Scott Aaronson, Xinyi Chen, Elad Hazan, and Satyen Kale.