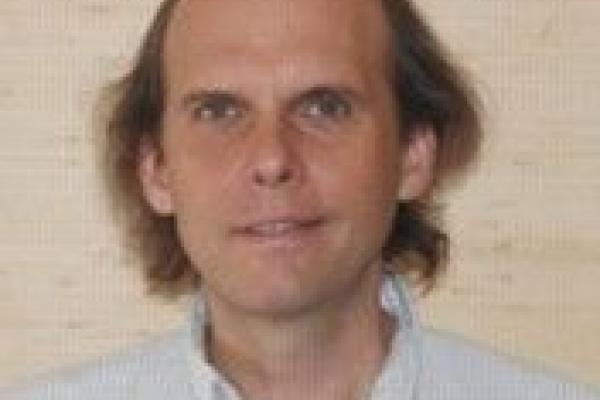
Retention of Science, Technology, Engineering, and Mathematics students is a critical national problem. Introductory physics, mathematics, and chemistry classes play a key role in the retention of these students. This talk will first explore retention through survival analysis to show the critical role of time in understanding retention. Machine learning algorithms including logistic regression, decision trees, and random forests are then applied to understand the variables important in predicting retention through the first year of college. This analysis will identify being a successful student in high school and arriving on campus “calculus-ready” as critical predictors of success. The student’s progression through the network of introductory science and mathematics courses is then explored. Machine learning algorithms are applied to understand a student’s risk factors as they matriculate from Calculus 1 and Chemistry 1 through Physics 1 and Physics 2. This will show students who matriculate through the network along different paths have different risk factors and chances of success.